Innovation is the key to a more interesting future.
Product-driven R&D
Exploratory R&D
Product-driven R&D
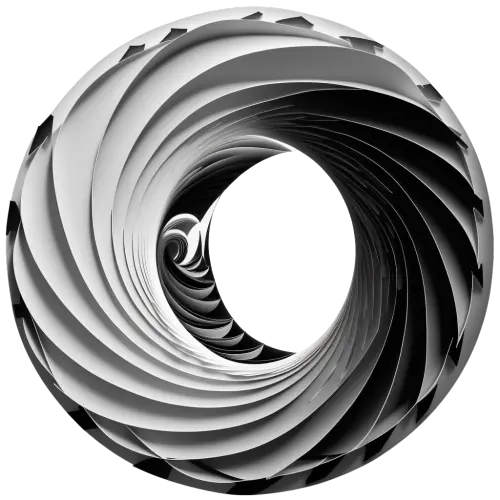
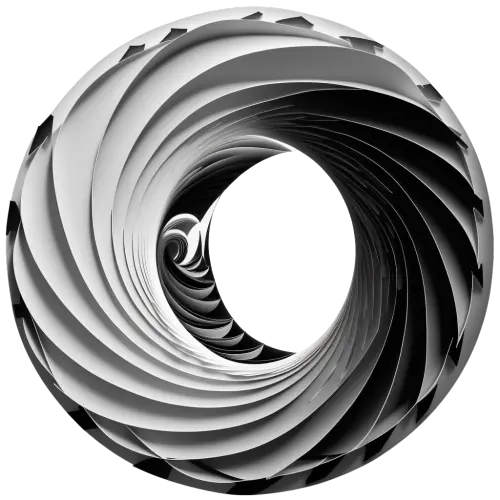
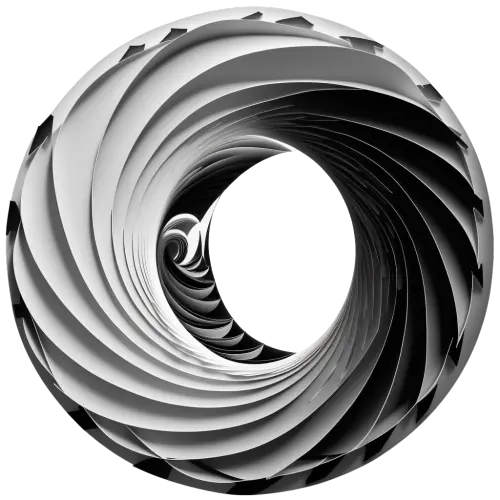
Trading
Ever been to a sporting stadium and seen the crowd suddenly wave their arms at the same time? Or, applause in an auditorium where the claps go from randomness to everyone clapping at the same time? That is synchrony in human behavior which is easy to identify with our visual and auditory senses. This type of synchrony is also occurring in the financial markets - but has been impossible to directly observe since we have never had data from mass social trading. However, with the markets now being open to an unprecedented number of individuals who are freely discussing their trades on social platforms, HerdForce can detect the synchrony in financial markets by analyzing this social behavior. The implication - a completely new way to solve trade discovery.
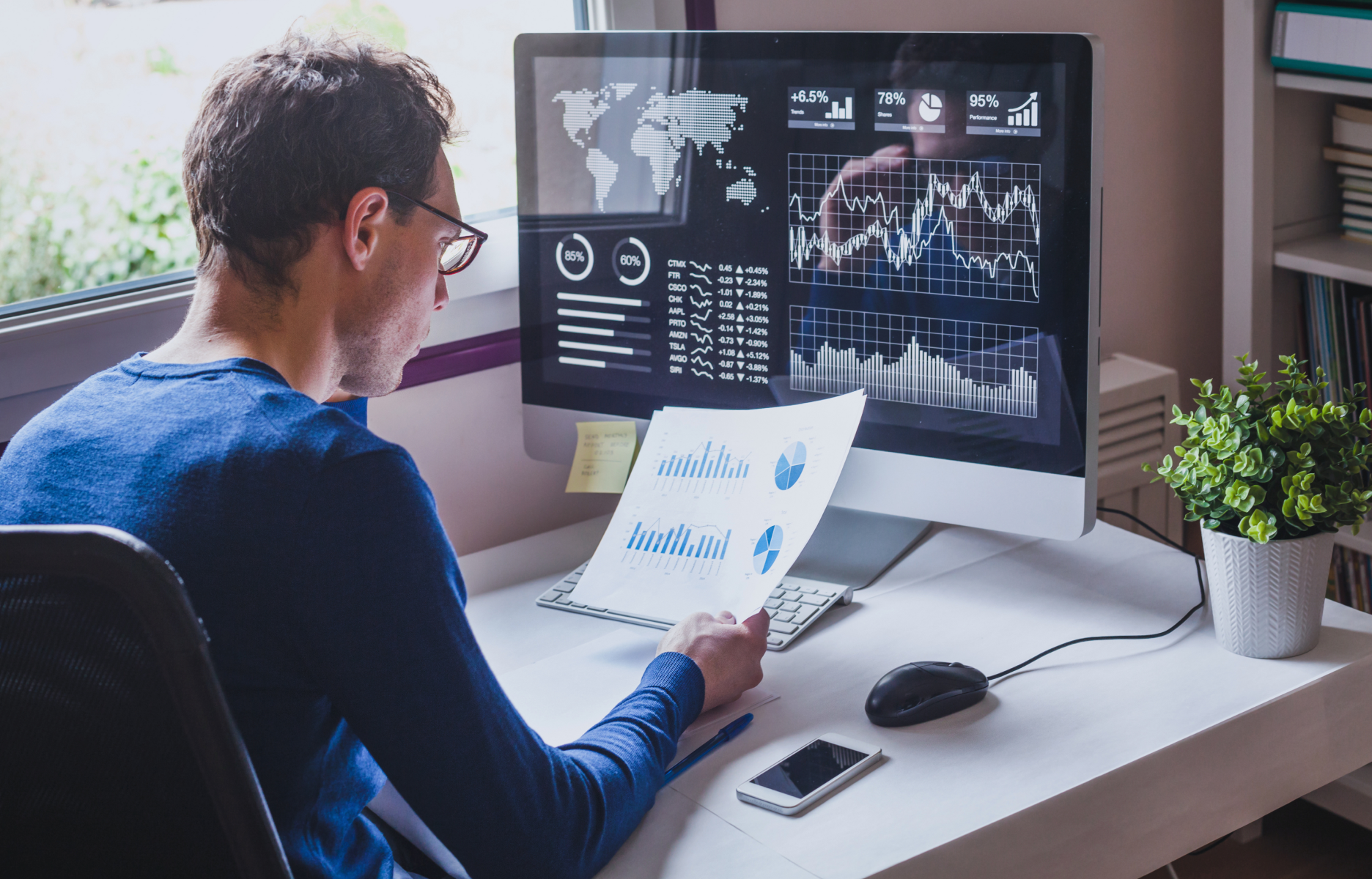
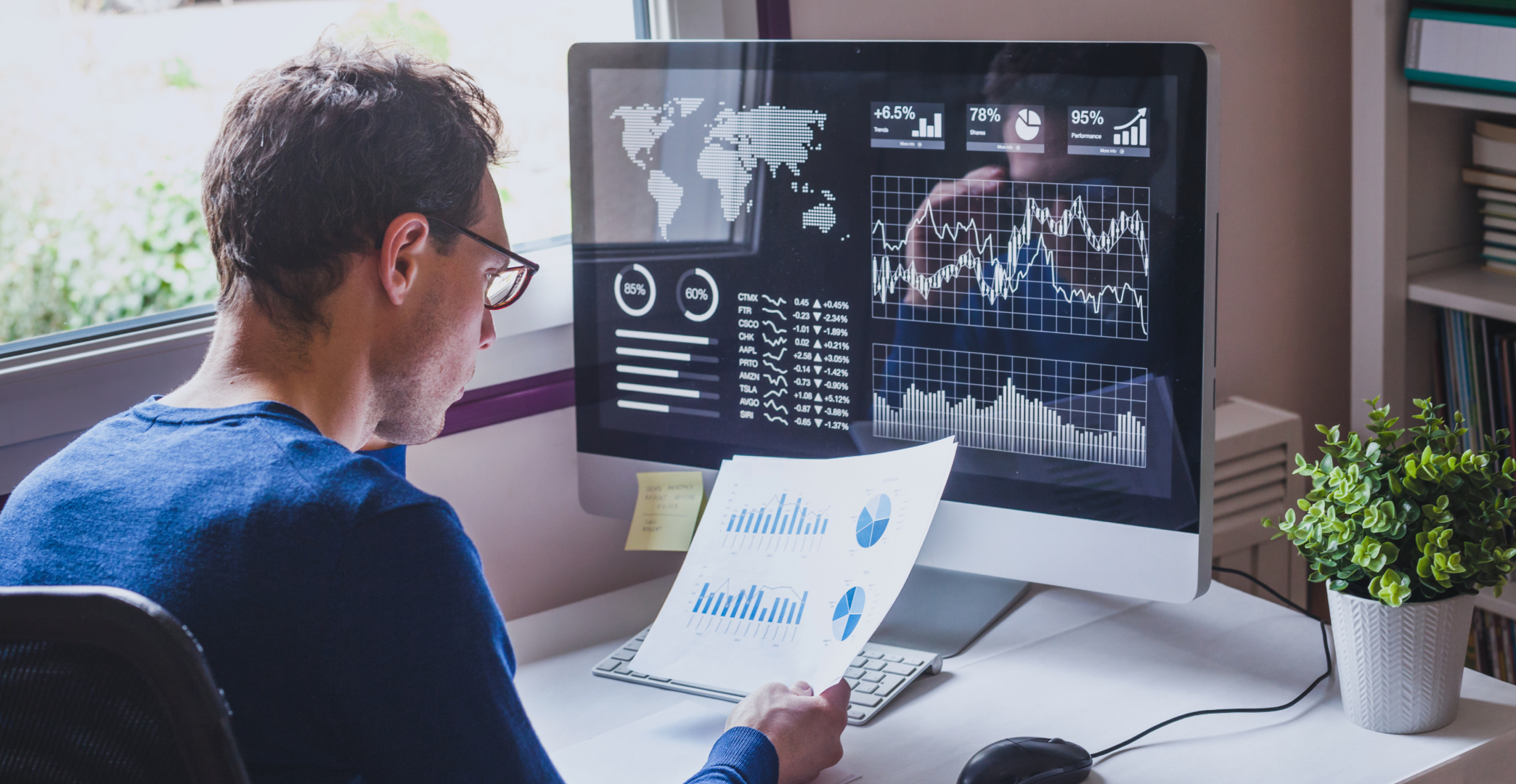
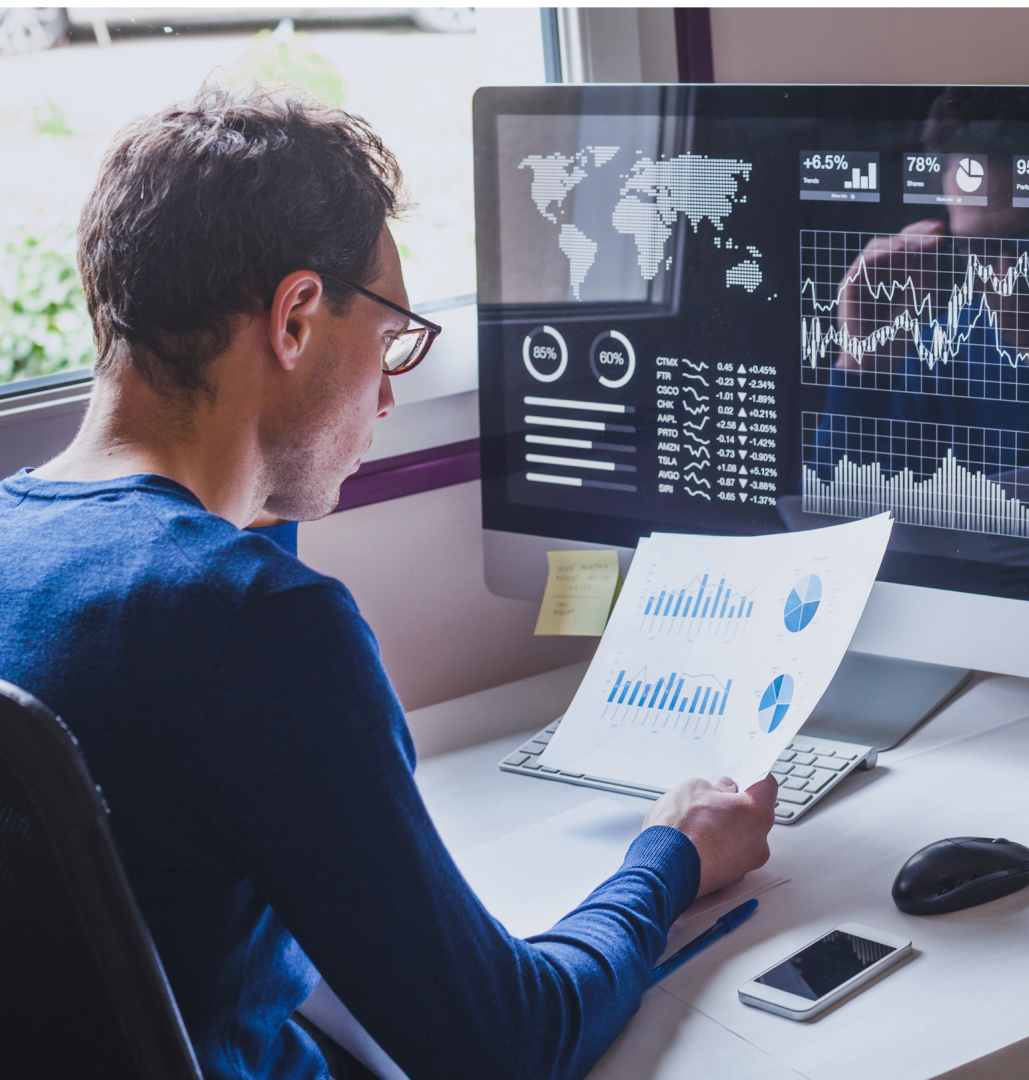
Investing
If everyone is now an investor, why are so few people good at fundamental analysis - picking companies by analyzing financial statements? Despite greatly improved access to markets, data, and multiple valuation approaches, very little progress has been made on tools that inspire sense-making of the data. Existing tools have converged on the kitchen sink approach - throwing every metric at the user, thereby creating more confusion rather than improving clarity. We are building an opinionated next-generation investing tool that unifies the central tenets of value and growth investing, while placing it within the context of current macroeconomic conditions. Stay tuned!
Exploratory R&D
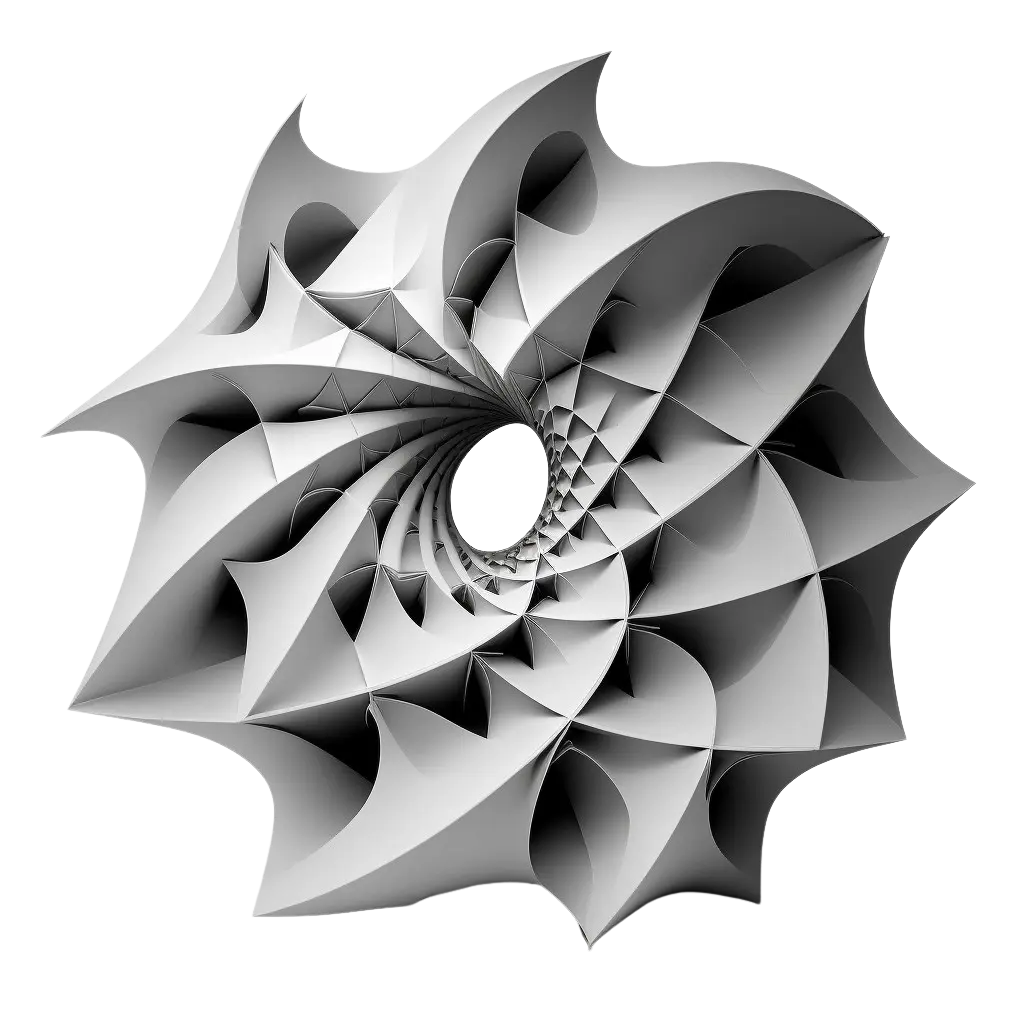
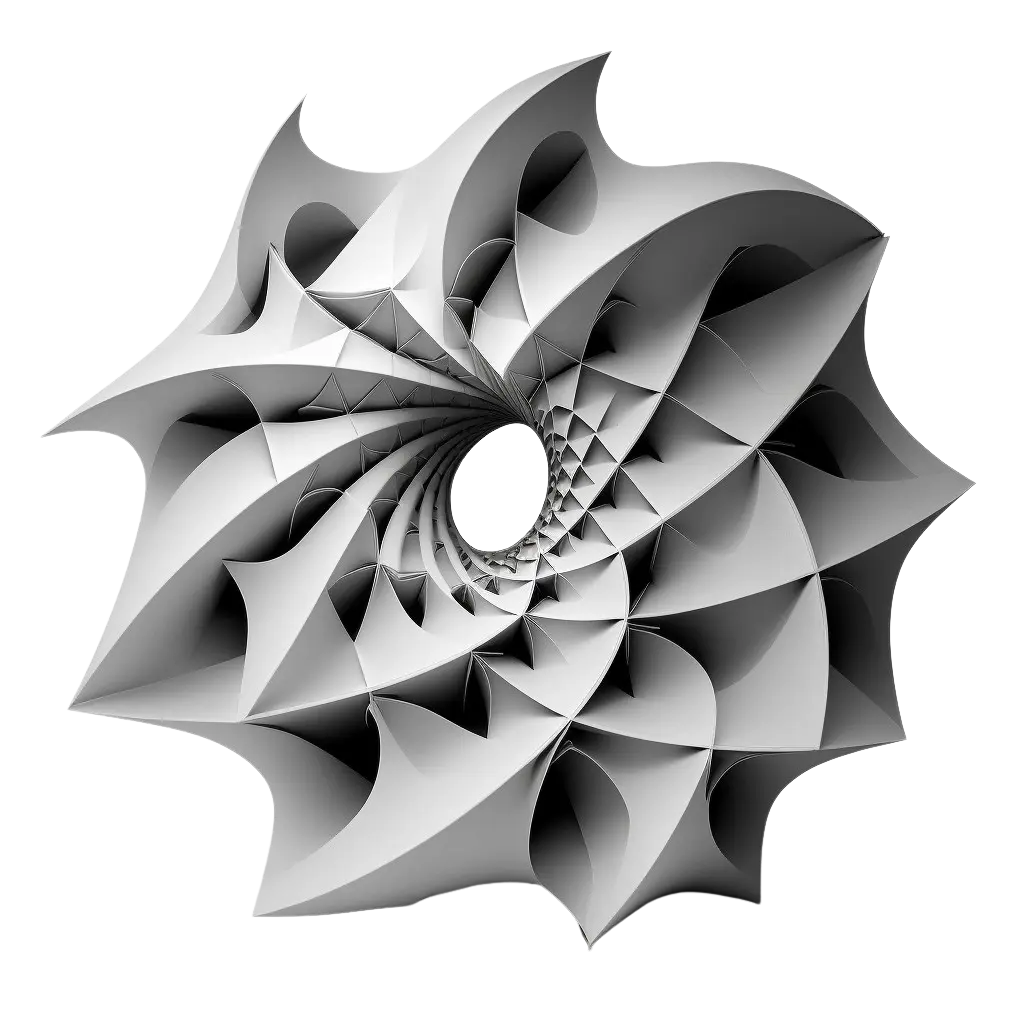
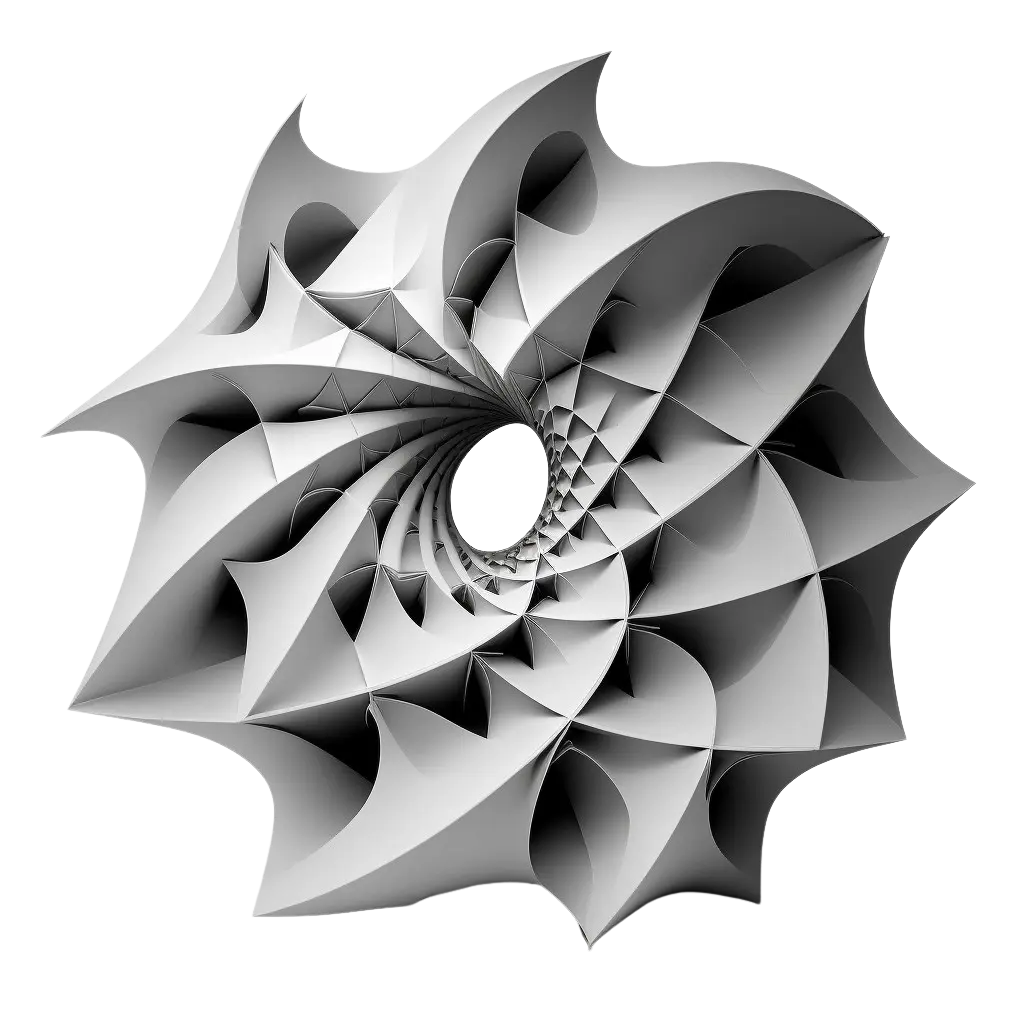
Reinforcement learning
Reinforcement learning (RL) is one of the most interesting areas of machine learning with deep connections to the literature on human learning. In the RL paradigm, agents learn through trial and error by interacting with an environment which provides delayed rewards associated with actions as opposed to explicit feedback. The goal in reinforcement learning is to learn state-action pairs such that actions maximize cumulative rewards over time.
There have been important breakthroughs in the academic literature which have pushed forward the state-of-the-art in applying reinforcement learning to games such as Go, which until quite recently was thought to be beyond the capacity of artificial intelligence systems. We are taking core ideas from such recent breakthroughs, and applying them in new ways, which we believe will lead to product innovation in novel application areas.
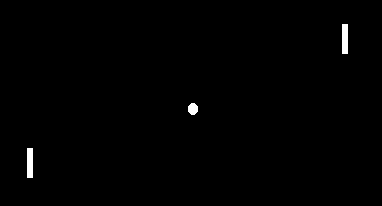
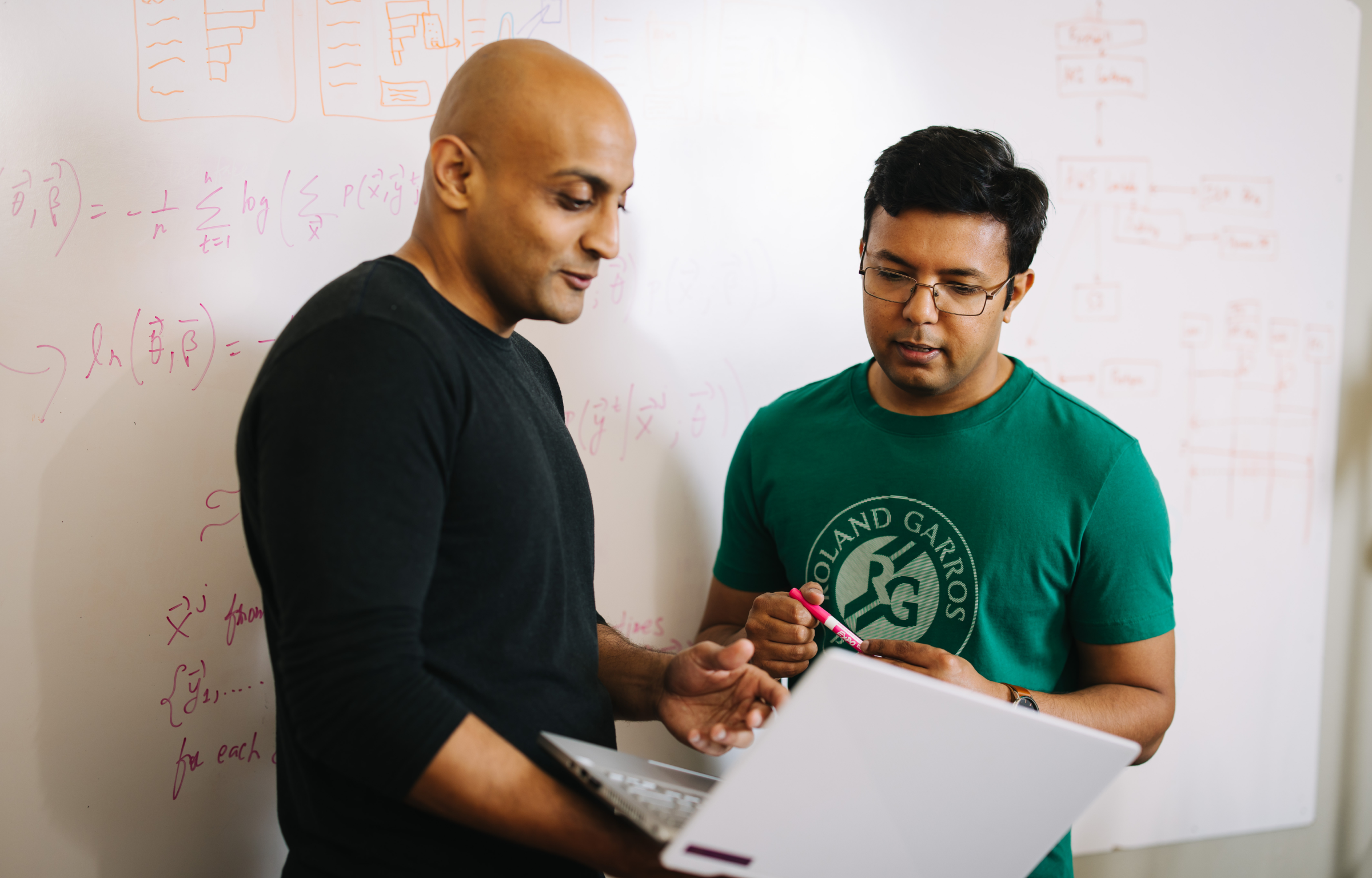
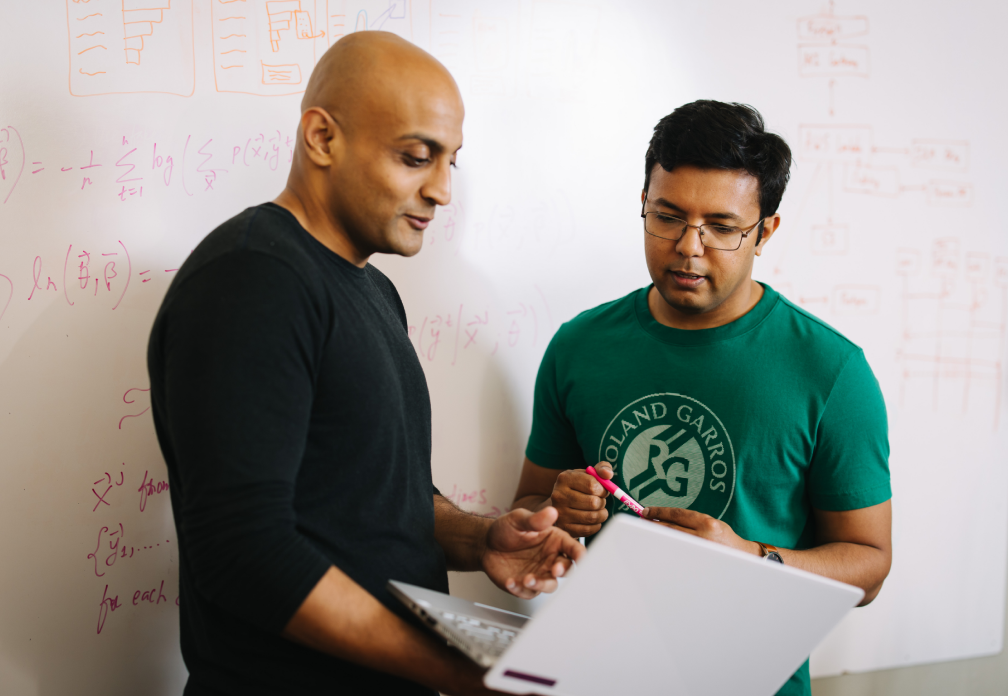
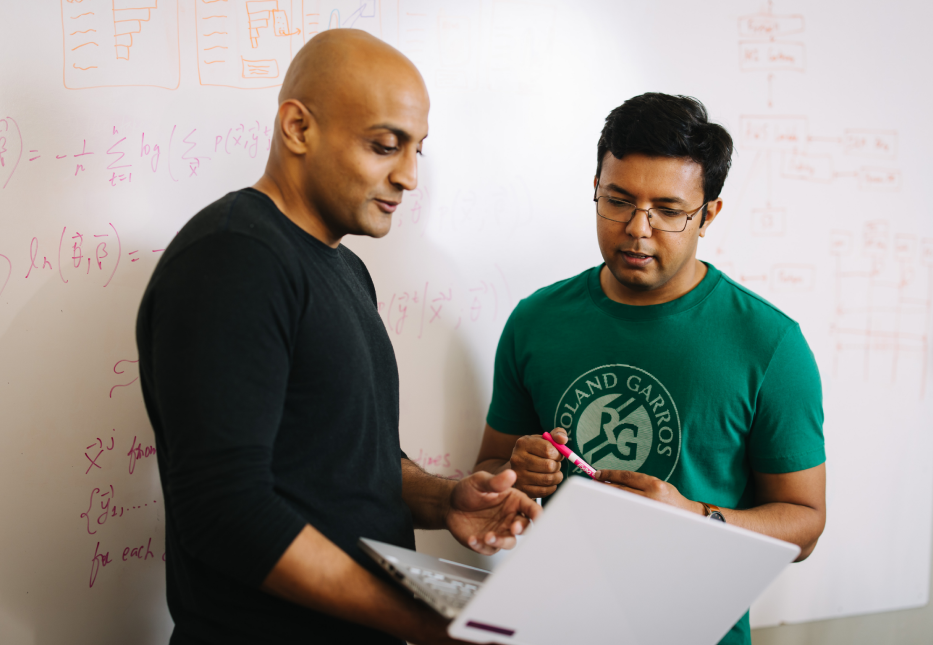